Zero to Autonomous
Last weekend DIYRobocars held the biggest race of its short history. We raced our 1/10th scale cars (and smaller) at ThunderHill Raceways as part of the Self Racing Cars event where some raced full sized cars and one go-cart on the 2 mile ThunderHill West track. Among the 3 classes of cars at the event between Self Racing Cars and DIYRobocars, I am the track master for what may be the most active track at the event, the 1/10th scale competition. Our modest 60 meter long track laid out with tape in the parking lot had 12 cars of which 7 completed fully autonomous laps. While all used vision the cars were based upon 5 different software stacks (2 based on OpenCV, one on a depth camera and 2 different CNN implementations). While most racers are from the greater Bay Area, we had racers join us from all over North America including Miami, Toronto and San Diego.
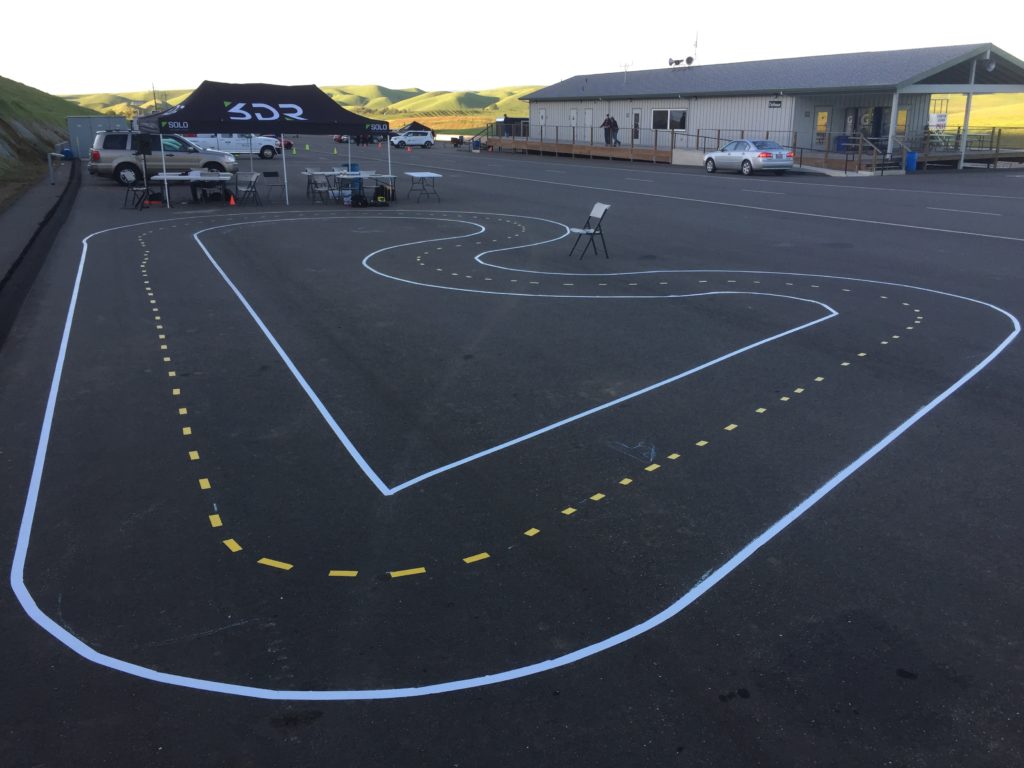
After this huge milestone it is worth reflecting on the short time that the DIYRobocars league has been in existence. The 1/10th leave kicked off with a hackathon organized by Chris Anderson at Carl Bass’ Warehouse on November 13 2016. As I look back on the last 4.5 months it is amazing what we have accomplished. Here are some stats:
- 23 total cars built
- 15 cars have raced
- 10 cars have completed an autonomous lap
- 21s – Fastest autonomous time
- 8s – Fastest Human time.
- And there is much more to come…
In addition times have consistently improved even though we have added new cars and racers as can be seen in the chart below
Compared to Self Racing Cars (full sized cars) at ThunderHill, our little cars did exceptionally well especially considering many of the full sized car projects have been running for years. Even with all of their funding, only 4 full-sized cars were able to run autonomous laps and not one was able to make a lap with vision only.
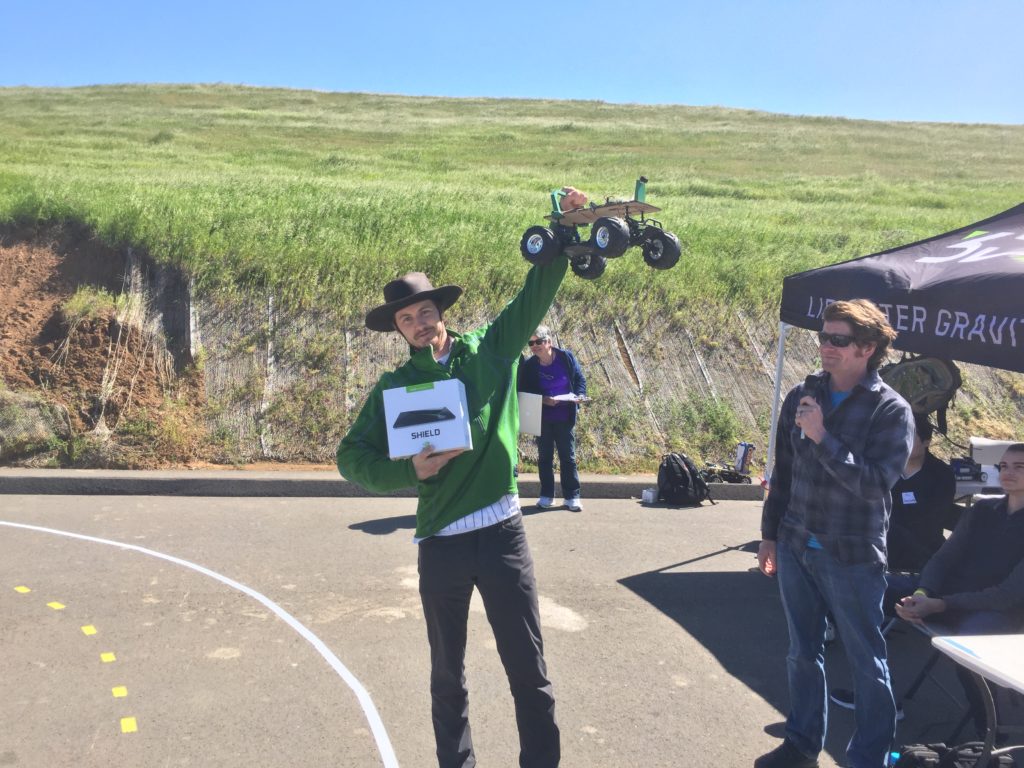
How was the 1/10th scale track able to demonstrate so much success in a shorter time? I am not totally certain, but I suspect two things are the primary causes:
First, the cost of failure is zero – The amount of caution required for a the development of an autopilot for a full size car must greatly hamper speed of innovation. With 1/10 we are able to take risks and test anywhere and move fast.
Second is less obvious – What makes DIYRobocars special is that it is a collaborative league. I cannot express how unusual this feels. While we are all competitors, we share our code, our secrets for winning and brainstorm with our competitors how to make our cars faster. Fierce competitors one moment are looking to merge code bases the next. In the larger car league, many cars are sponsored by competing companies. There is much less sharing and collaboration which puts the brakes on innovation.
While the last 4 months have been great, I also look towards all we accomplish in the next 4. While many designs will be refined and lap-times will drop, the next big step is to mix up the format to tackle the next set of technical challenges. Our next big rule and format change is to incorporate is wheel-to-wheel racing which introduces a new set of technical problems. More on the rule changes in the coming weeks.